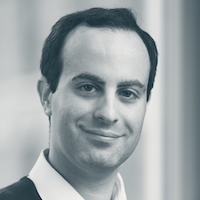
I am Chief AI Scientist at Databricks, where I lead the research team. I arrived via Databricks' $1.3B acquisition of MosaicML, where I was a member of the founding team. I completed my PhD in computer science at MIT in 2023. You can find my research on Google Scholar. I live in New York City, and I travel frequently to San Francisco and Washington, DC.
Research
Mosaic Research at Databricks
At Databricks, we develop more efficient ways to build and customize AI systems. We work on topics ranging from natural lanugage processing and LLMs to computer vision and multimodal models. We share this work with the community in the form of blog posts, open source resositories (like Composer, Streaming, LLM Foundry, and MegaBlocks), and open source models (like DBRX and MPT).
The Lottery Ticket Hypothesis
My main line of research during my PhD was on my lottery ticket hypothesis. This line of research focuses on understanding how large neural networks need to be to train in practice. We have long known that we can make neural networks much smaller after they have been trained. In this line of work, I showed that they can be equally small for much or all of training. This research has revealed new insights into how neural networks learn and offered opportunities for practical efficiency improvements.
The Science of Deep Learning
More broadly, I am interested in empirically understanding the behavior of practical neural networks. For all the extraordinary AI advances that neural networks have enabled in recent years, our understanding of how and what they learn remains limited. I study these questions from a scientific perspective, posing hypotheses and performing large-scale experiments to empircally evaluate them. I think we can build better and AI systems - and do so more efficiently - if we understand how neural networks learn, and I think the best way to do so is to study the real artifacts that have made us so excited about AI in the first place.
Technology Policy
I spend a portion of my time working on technology policy. In this capacity work closely with lawyers, journalists, and policymakers on topics related to AI. I currently work with the OECD to implement the AI Principles that we developed in 2019. I previously served as the inaugural Staff Technologist at the Center on Privacy and Technology at Georgetown Law, where I contributed to a landmark report on police use of face recognition (The Perpetual Lineup) and co-developed a course on Computer Programming for Lawyers with Prof. Paul Ohm.